By Angie Schmitt

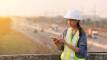
Georgia Tech Professor Yi-Chang (James) Tsai recently helped Georgia Department of Transportation (GDOT) complete a survey using AI. Using GPS and cell phones mounted in GDOT vehicles, the team was able to analyze 22,000 road signs around potentially dangerous road curves.
“Instead of taking one or two years,” said Tsai at the Transportation Research Board webinar: Deploying AI Applications for Asset Management, “it can take one or two days to get it done.”
The project was aimed at improving safety at road curves which are a risk factor for crashes. Condition-appropriate warning signs can reduce crash rates substantially. To improve safety, Georgia wanted to know where the signs were missing or inadequate.
Tsai and his team used AI technology to catalogue sign data from 18,000 miles of roadways for GDOT.
Leading researchers are still fine tuning the process of these lightning-quick, AI-assisted automated asset management analyses. But it is fast becoming the norm. Another panelist, Ken Yang, Senior Systems Engineer at AECOM, reported that 80 percent of state DOTs now use AI to conduct pavement inspections.
In 2017, Yang was able to help the Texas DOT complete a survey of pavement conditions on its entire interstate system using LiDAR technology and AI. The team gathered raw data on pavement cracks and conditions using LiDAR technology that was mounted on specially fitted agency vehicles. According to Yang, LiDAR scanning allowed the agency not only to gather data day or night, but also improve accuracy and eliminate visual noise.
The team used Google’s Vertex AI to analyze raw data. Google’s Auto Machine Learning “object tracking” and “classification” features helped them — with some careful tweaking — categorize pavement conditions on a scale of one to 10. Yang told attendees it has reduced the time needed to complete the project by 30 to 70 percent.
Gathering huge amounts of detailed data and using it to produce comprehensive maps that can inform decision making has never been quicker or easier.
Yaw Adu-Gyamfi, an assistant professor at the University of Missouri-Columbia, discussed how he built a model to analyze, not just pavement conditions, but signs and street markings throughout Jefferson City. The team used a GoPro camera and Ouster LiDAR to capture reflexivity data (such as signs and street marking conditions). Adu-Gyamfi’s three-part machine learning model could detect a pavement marking, segment it and give its condition. The team used Firebase and fastAPI as the back-end software and React JS as the front-end, with the AI model serving as the intermediary, he said.
Adu-Gyamfi reported his model was able to detect and categorize sign data with 94 percent accuracy and pavement conditions with 74 percent accuracy. Though the team is still fine tuning their approach, they were able to analyze the infrastructure conditions on the city’s 250 miles of roadway in only three days.